Why Traditional Data Security Measures Aren’t Enough for AI-Powered Businesses
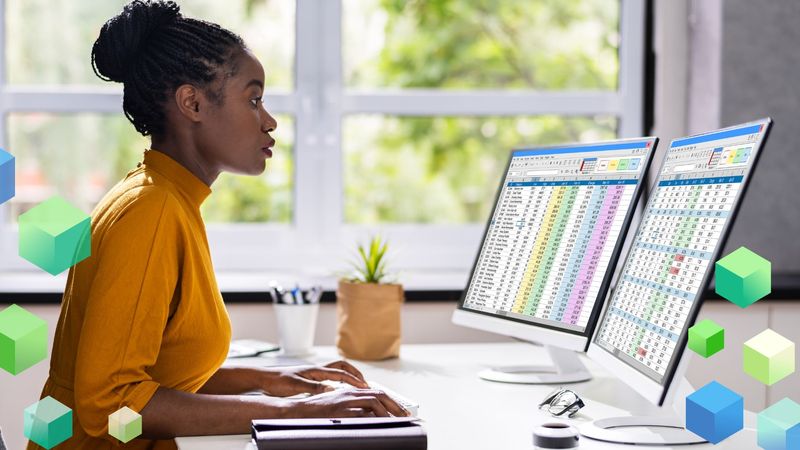
In today’s rapidly evolving digital landscape, artificial intelligence (AI) has become a cornerstone of innovation. Businesses across various industries are leveraging AI to enhance decision-making, improve customer experience, and streamline operations. However, as AI systems become more integrated into business infrastructures, traditional data security measures are proving to be insufficient.
AI systems come with their unique challenges, and the data they process often requires more robust protection than what traditional security models offer. Here’s why traditional data security measures aren’t enough for AI-powered businesses and what can be done to address these challenges.
Volume, Variety, and Velocity of Data
AI systems thrive on vast amounts of data—often including sensitive, proprietary, or personally identifiable information (PII). Traditional security measures like firewalls and encryption were designed to protect more static, centralized data systems. However, AI deals with dynamic data that’s continuously collected, processed, and analyzed in real-time from multiple sources, often in large volumes.
Solution: Modern AI-powered businesses must adopt more advanced data security solutions, such as continuous data monitoring, behavior-based anomaly detection, and real-time threat identification. These systems should evolve to monitor and protect the growing diversity and velocity of data that AI processes.
Data Integrity and Model Corruption
AI models are trained on data, and any compromise in the integrity of that data can significantly affect the results produced by the AI. Traditional data security measures focus on protecting data from unauthorized access or theft, but they may not prevent subtle manipulation of data used for training AI models. A corrupted model can lead to incorrect predictions or decisions, causing financial or reputational harm to businesses.
Solution: Businesses should implement robust data integrity verification mechanisms. Blockchain technology, for instance, can offer a tamper-proof way to track changes and ensure that only verified, accurate data is used in AI training. Model monitoring tools can also detect and alert businesses if the AI model behaves abnormally due to corrupted data.
Increased Attack Surface
AI systems typically interface with many external data sources, APIs, and third-party applications, creating a much larger attack surface than traditional business systems. Hackers can exploit these connections to inject malicious data or gain unauthorized access to sensitive data.
Traditional security measures that focus on securing individual systems or endpoints are no longer sufficient. The interconnected nature of AI systems means that a breach in one area can potentially expose the entire system.
Solution: A zero-trust security framework is crucial for AI-powered businesses. This approach involves continually verifying every connection, device, and user, regardless of whether they are inside or outside the network. AI security systems should also implement strong encryption protocols, multi-factor authentication, and secure APIs to minimize vulnerabilities.
Adversarial Attacks
Adversarial attacks are unique to AI systems and involve the manipulation of input data to fool an AI model into making incorrect predictions or decisions. These attacks are not a concern in traditional data security models but pose a significant risk to AI businesses that rely on accurate AI outputs.
For instance, in image recognition systems, adversarial attackers can slightly alter an image in a way that causes the AI model to misclassify it, even though the alteration is imperceptible to the human eye. These attacks can have severe consequences in industries like healthcare, finance, and autonomous driving.
Solution: AI businesses need to integrate adversarial training, a technique where the model is trained on both regular and adversarial examples. This prepares the AI to recognize and defend against manipulated inputs. Additionally, AI models must undergo regular security audits to ensure that they are resilient against adversarial attacks.
Data Privacy Concerns
As AI systems process vast amounts of personal and sensitive information, businesses must comply with stringent data privacy regulations such as GDPR, CCPA, and others. Traditional security frameworks were often built without considering these modern privacy laws, leading to potential compliance issues.
Moreover, AI systems may inadvertently expose sensitive data if not properly secured. For example, machine learning models could retain identifiable information about individuals from the training data, raising privacy concerns.
Solution: AI-powered businesses must adopt privacy-by-design principles, ensuring that data privacy is built into the AI system from the ground up. Techniques like data anonymization, differential privacy, and federated learning can help in securing sensitive data while still allowing AI systems to function effectively.